mirror of
https://github.com/immich-app/immich.git
synced 2025-01-25 05:02:46 +01:00
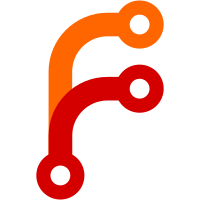
* modularize model classes * various fixes * expose port * change response * round coordinates * simplify preload * update server * simplify interface simplify * update tests * composable endpoint * cleanup fixes remove unnecessary interface support text input, cleanup * ew camelcase * update server server fixes fix typing * ml fixes update locustfile fixes * cleaner response * better repo response * update tests formatting and typing rename * undo compose change * linting fix type actually fix typing * stricter typing fix detection-only response no need for defaultdict * update spec file update api linting * update e2e * unnecessary dimension * remove commented code * remove duplicate code * remove unused imports * add batch dim
60 lines
2.1 KiB
Python
60 lines
2.1 KiB
Python
from typing import Any
|
|
|
|
from aiocache.backends.memory import SimpleMemoryCache
|
|
from aiocache.lock import OptimisticLock
|
|
from aiocache.plugins import TimingPlugin
|
|
|
|
from app.models import from_model_type
|
|
from app.models.base import InferenceModel
|
|
|
|
from ..schemas import ModelTask, ModelType, has_profiling
|
|
|
|
|
|
class ModelCache:
|
|
"""Fetches a model from an in-memory cache, instantiating it if it's missing."""
|
|
|
|
def __init__(
|
|
self,
|
|
revalidate: bool = False,
|
|
timeout: int | None = None,
|
|
profiling: bool = False,
|
|
) -> None:
|
|
"""
|
|
Args:
|
|
revalidate: Resets TTL on cache hit. Useful to keep models in memory while active. Defaults to False.
|
|
timeout: Maximum allowed time for model to load. Disabled if None. Defaults to None.
|
|
profiling: Collects metrics for cache operations, adding slight overhead. Defaults to False.
|
|
"""
|
|
|
|
plugins = []
|
|
|
|
if profiling:
|
|
plugins.append(TimingPlugin())
|
|
|
|
self.should_revalidate = revalidate
|
|
|
|
self.cache = SimpleMemoryCache(timeout=timeout, plugins=plugins, namespace=None)
|
|
|
|
async def get(
|
|
self, model_name: str, model_type: ModelType, model_task: ModelTask, **model_kwargs: Any
|
|
) -> InferenceModel:
|
|
key = f"{model_name}{model_type}{model_task}"
|
|
|
|
async with OptimisticLock(self.cache, key) as lock:
|
|
model: InferenceModel | None = await self.cache.get(key)
|
|
if model is None:
|
|
model = from_model_type(model_name, model_type, model_task, **model_kwargs)
|
|
await lock.cas(model, ttl=model_kwargs.get("ttl", None))
|
|
elif self.should_revalidate:
|
|
await self.revalidate(key, model_kwargs.get("ttl", None))
|
|
return model
|
|
|
|
async def get_profiling(self) -> dict[str, float] | None:
|
|
if not has_profiling(self.cache):
|
|
return None
|
|
|
|
return self.cache.profiling
|
|
|
|
async def revalidate(self, key: str, ttl: int | None) -> None:
|
|
if ttl is not None and key in self.cache._handlers:
|
|
await self.cache.expire(key, ttl)
|